Businesses are increasingly turning to artificial intelligence (AI) to enhance customer experiences through personalized recommendations. This article explores how AI can revolutionize product recommendations and outlines best practices to develop effective AI-driven recommendation engines.
Research indicates that AI algorithms significantly impact consumer behavior, particularly in e-commerce and streaming platforms, by providing personalized suggestions that closely align with individual preferences (MTR Marketing, 2024). These tailored experiences not only increase customer satisfaction but also drive engagement and retention rates, leading to increased revenue for businesses.
The effectiveness of AI in generating product recommendations is attributed to its ability to analyze vast amounts of data quickly and accurately. By leveraging machine learning, AI can identify patterns and predict future behaviors based on historical data, allowing businesses to offer more relevant products and services to their customers (CMSWire, 2023). This capability is critical in today’s competitive market, where customer expectations are continuously evolving and instantaneous, personalized interactions are the norm.
To maximize the potential of AI-driven recommendation engines, businesses should consider several best practices. These include ensuring data quality, leveraging diverse data sources, integrating feedback loops, and maintaining transparency with customers about how their data is used (SpringerLink, 2020). High-quality, clean data is fundamental for training accurate AI models, while incorporating a variety of data sources enriches the recommendation system, making it more comprehensive.
Integration of feedback loops helps the AI system to continually learn and adapt from user interactions, refining its recommendations over time. Transparency fosters trust, allowing customers to feel more comfortable with personalization efforts, knowing their privacy is protected and their data is responsibly managed.
In Boston and beyond, the potential for AI to transform customer experiences through personalized recommendations is immense. Companies that harness this technology effectively will likely see substantial benefits in customer loyalty and business growth. This article delves deeper into the nuances of AI-driven recommendation systems, discussing the intricacies of their development and implementation.
Understanding AI and Recommendation Systems
The success of AI-driven recommendation systems hinges on their ability to tailor user experiences with high precision. AI plays a pivotal role in personalization by analyzing user data to generate recommendations that resonate with individual tastes and behaviors. This process enhances the customer journey, making interactions with platforms more engaging and satisfying.
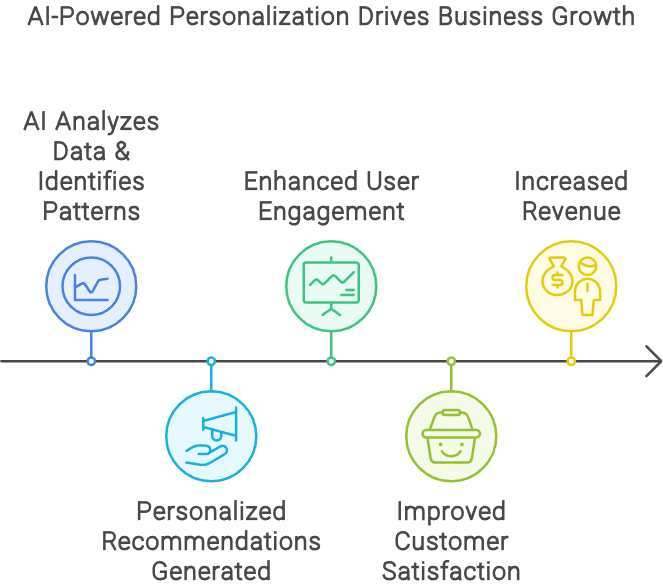
The Role of AI in Personalization
AI recommendations utilize powerful machine learning algorithms to sift through extensive datasets and identify patterns that might elude human analysts. These personalization algorithms can predict what products or services a user might be interested in, thus bringing value to both customers and businesses.
- Enhanced User Engagement: By presenting users with relevant content, AI-driven systems keep users engaged for longer periods.
- Improved Customer Satisfaction: Personalized suggestions lead to higher customer satisfaction as users feel their preferences are understood and catered to (MTR Marketing, 2024).
- Increased Revenue: Tailored recommendations often result in higher conversion rates, which directly increase revenue for businesses (MTR Marketing, 2024).
Types of Recommendation Systems
Recommendation systems are broadly categorized based on their operational techniques:
- Collaborative Filtering:
This type operates by analyzing user behavior and preferences to recommend items that similar users have shown interest in. It relies heavily on user interaction data such as ratings, clicks, or purchase history. One common issue is the "cold start" problem, where the system struggles to make accurate recommendations for new users due to the lack of prior data (CMSWire, 2023). - Content-Based Filtering:
This system recommends items similar to ones the user previously liked or engaged with, based on item attributes and user preferences. It effectively eliminates the cold start problem since it does not rely on other users' data to generate recommendations but might struggle to give serendipitous recommendations (CMSWire, 2023). - Hybrid Systems:
Hybrid recommendation systems combine collaborative and content-based approaches to maximize the strengths of both methods while mitigating their weaknesses. These systems are increasingly being adopted as they offer more accurate and diverse product recommendations (SpringerLink, 2020).
Best Practices for AI Recommendation Systems
To build effective AI-driven recommendation engines, it is essential to incorporate certain best practices:
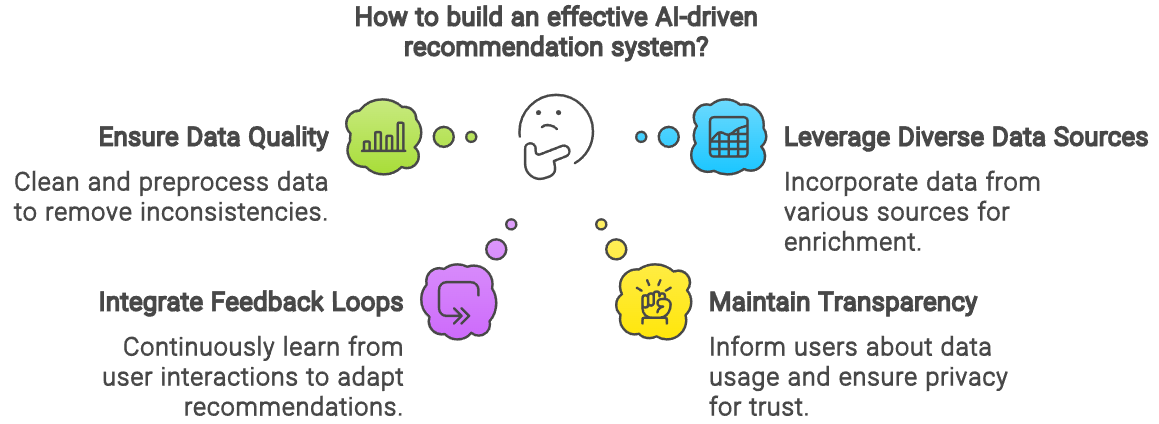
- Ensuring Data Quality: High-quality data is pivotal for training reliable AI models. Data should be cleaned and preprocessed to remove any inconsistencies or inaccuracies.
- Leveraging Diverse Data Sources: Incorporating data from various sources, such as user interactions, social media, and demographic information, can enrich the recommendation process.
- Integrating Feedback Loops: Continuous learning from user interactions allows the recommendation system to adapt and refine its suggestions. This iterative process helps maintain the relevance of recommendations.
- Maintaining Transparency: Informing customers about how their data is used and ensuring their privacy is a crucial aspect. Transparency builds trust and comfort among users, which can lead to higher acceptance of personalized content.
In Conclusion
The ability to provide AI recommendations that are both accurate and personalized is transforming how businesses operate in the digital landscape. Companies that adeptly navigate the complexities of AI and personalization algorithms will likely foster stronger customer loyalty and achieve significant growth.
By following these best practices, businesses can harness the full potential of AI-driven recommendation systems, staying competitive in an ever-evolving market. As you explore further, more articles on our site delve into the intersection of AI, personalization, and customer experience, offering a comprehensive understanding of this dynamic field.
References
- MTR Marketing. (2024, January 15). How (and Why) to Leverage AI for Personalized Marketing Strategy. Retrieved from https://www.mtrmarketing.com/blog/how-and-why-to-leverage-ai-for-personalized-marketing-strategy
- CMSWire. (2023, June 29). AI & Data Analytics: Redefining Customer Personalization. Retrieved from https://www.cmswire.com/customer-experience/how-ai-and-data-analytics-drive-personalization-strategies/
- SpringerLink. (2020, November 1). Artificial intelligence in recommender systems. Retrieved from https://link.springer.com/article/10.1007/s40747-020-00212-w